MAY 14, 2025
27 min listen
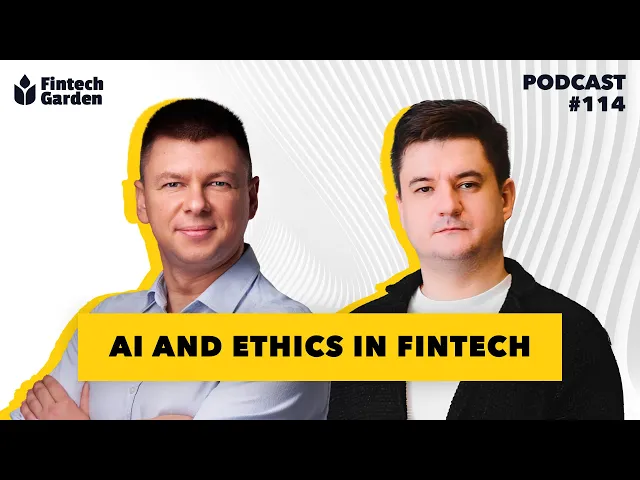
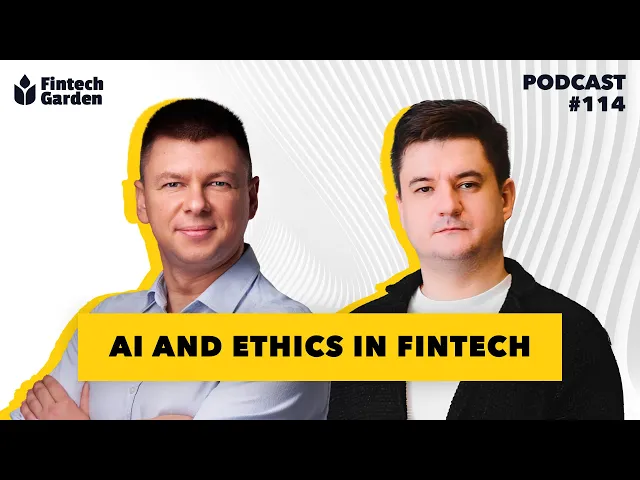
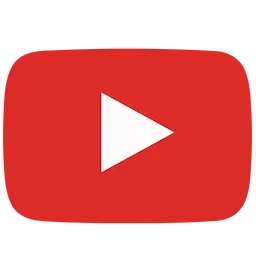
Hosts
Tune in to the Full Podcast Episode Below
How Bias in Machine Learning Affects Credit Scoring
In the latest episode of the Fintech Garden podcast by DashDevs, Igor Tomych and Dumitru Condrea explore a pressing topic in modern financial technology: the ethical risks associated with using artificial intelligence and machine learning in credit scoring.
As fintech companies increasingly rely on automated decision-making systems, questions around fairness, transparency, and unintended discrimination are becoming more urgent. This episode provides a grounded, real-world look into how these systems can behave unpredictably—even unfairly—despite being built by human hands.
When AI Gets It Wrong: Real-World Examples
The discussion opens with two practical cases. Two individuals, both with identical financial profiles—same income, employment status, and credit history—received opposite decisions: one was approved for credit, the other rejected. The only difference? How the underlying machine learning model segmented them.
These examples highlight a growing problem: AI systems, often seen as neutral, can replicate or even amplify human bias if not built and monitored carefully.
How Bias Enters AI Credit Models
Igor and Dumitru explain how modern credit scoring algorithms are created and where ethical issues emerge during the design and training process:
- Bias in training data: Historical data may reflect societal biases, which are then learned and reproduced by AI models.
- Regional and cultural segmentation: Models trained on one region may behave very differently when applied in another, sometimes unfairly penalizing users.
- Conflicting priorities: Many AI systems are optimized for business KPIs such as default rates or approval thresholds, rather than fairness or transparency.
They argue that the root of the problem often lies not in bad intent, but in unquestioned assumptions and overlooked variables within the model training and validation process.
The Need for Human Oversight and Ethical Design
To mitigate these risks, the conversation turns toward actionable strategies for teams building or deploying credit scoring systems:
- Ensure models are auditable and explainable
- Regularly test scoring logic for demographic or regional bias
- Engage multidisciplinary teams—combining product, data science, compliance, and business functions—to align model outcomes with ethical standards
For markets operating under tighter regulatory scrutiny, these practices are not optional—they are essential.
Business Implications for Fintech Leaders
The episode concludes with a broader message for fintech founders, product owners, and AI leaders: ethics is not separate from strategy. How your product makes decisions impacts brand trust, user retention, and regulatory compliance.
Teams that fail to consider fairness risk reputational damage and missed opportunities for inclusive growth. Those that proactively address AI ethics build more resilient, trusted platforms.
Why This Matters
Credit scoring is one of the most influential applications of AI in fintech. When done well, it expands access. When done poorly, it excludes users unfairly. This episode of Fintech Garden challenges listeners to look beyond performance metrics and consider how their systems impact real people.
At DashDevs, we help fintech companies build credit products that are powerful, scalable—and responsible.
Listen to Episode 114 now and discover how to design AI systems that are both intelligent and ethical.
Hosts